Why Sigma?
Quality 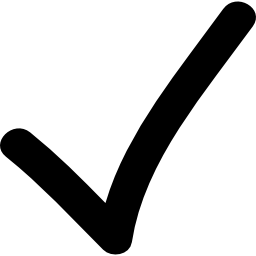
Your AI is only as good as its training data
Sigma’s high quality training data enables the development of the best AI there is.
We guarantee 98% accuracy using our QA methodology and tools. Need even higher? You tell us the accuracy you need, up to 99.99%, and we’ll hit it.
Accuracy, being a critical quality parameter, is not the only one, since quality is multidimensional and must be interpreted in relation to the AI targeted goals. Sigma’s comprehensive QA methodology combines the following parameters to measure and assure training data quality:
Accuracy:Â High data annotation accuracy is the result of three factors:
- Preventive Measures prevent annotation errors from happening. These include selecting the right human team, providing the team with necessary training, testing the team, reinforcing the training in the most error prone tasks, keeping a motivating working environment, designing user-friendly and intuitive tools, implementing active error minimization measures, automating processes, and optimizing the combination of ML-assisted tools and human annotators among others.
- Reactive Measures detect and fix errors and issues once they have occurred. Errors can be classified into three categories: Occasional errors, systematic errors and interpretation issues. Systematic errors and interpretation issues are responsible for the lack of annotation consistency, so there must be a strategy to detect, correct and provide feedback.
- Metrics measure and track the level of quality. It is usually necessary to combine several metrics to better understand the errors and/or the deficiencies in the data.
Volume of Data:Â If the data available is not sufficient to achieve the performance goals, the dataset does not meet the requirements. Sigma has the experience to help determining the optimal volume of training data.
Data Consistency:Â Similar to how children learn, artificial intelligence algorithms learn from examples (the training data). If the examples have errors or are not consistently annotated, the AI will not learn properly and will not work at its full potential. It is essential that all the data annotations have been done following the exact same annotation guidelines.
Domain Coverage:Â Training data has to represent the particular characteristics of the domain in which the resulting AI-based system is going to work. The closer the data is to the real working conditions, the better the performance of the system.
User’s Coverage: In a similar way to domain coverage, the training data has to properly represent the end-users in terms of parameters such as age-group, gender, level of education, race, and language/dialect. In the same way, data should take into account if the users are expected to be collaborative or non-collaborative.
Balance: No question that artificial intelligence is not affected by fatigue, friendship, emotion, sickness or any other human characteristic. However, this does not mean that AI will perform in a more objective way than humans. AI can be discriminatory or make mistakes if the training data is biased and does not represent reality in a balanced way.