Sigma’s Text Annotation Services
Improve your Natural Language Processing Models with high quality text annotations.
Our ML-assisted Tools can reduce annotation time and cost significantly.
Sigma has highly secure facilities to annotate confidential and PII data.
What is Text Annotation?
[zb_ziggy_bot]
Text Annotation for machine learning consists of associating labels to digital text files and their content. Text annotation converts a text into a dataset that can be used to train machine learning and deep learning models for a variety of Natural Language Processing applications.
Texts need to be enriched through the annotation process because natural language is complex and full of nuances. For example, the meaning of a sentence can be affected by the context, the relationship between words or phrases (coreference), the omission of a word or several words (ellipsis), etc.
A properly annotated text allows natural language processing algorithms learn to interpret texts and perform sophisticated tasks such as human-machine communication, intent estimation, sentiment analysis, language generation, machine translation, text classification, fake news detection, etc.
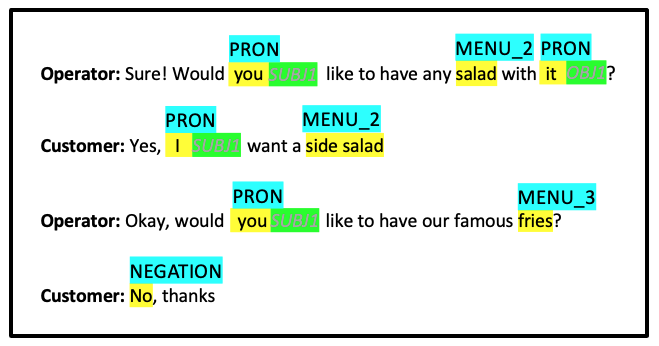
Text Annotation Services
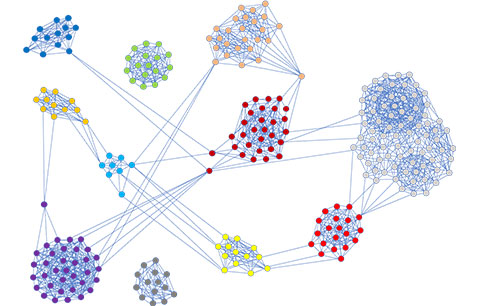
Text Classification
It consists of reading a written input and classifying it into a series of categories that have been previously defined.
For example, categories that describe the topic, or the language or dialect.
Correctly classified text datasets are used in Natural Language Processing to train automatic text classifiers, which are an excellent alternative to scale the process of structuring textual data.
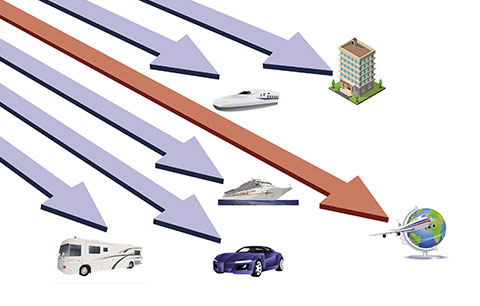
Intent Recognition
It is the process of reading a written input or listening to a voice recording and classifying it according to what the person wants to achieve.
Intent recognition is particularly useful in the call center area, chatbots and intelligent agents since it provides relevant information to improve customer service in sales, customer support, information search, etc.
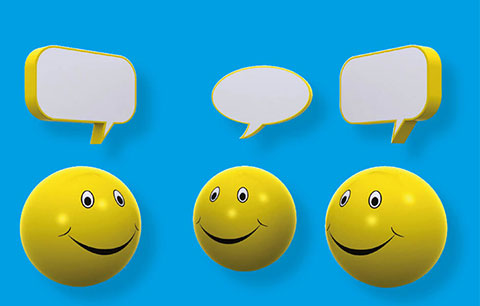
Sentiment Annotation
It is the process of determining if a text is perceived as positive, negative or neutral.
It helps gauge customers opinion, monitor brand/product reputation, customer experience and needs, social media, chatbots, etc.
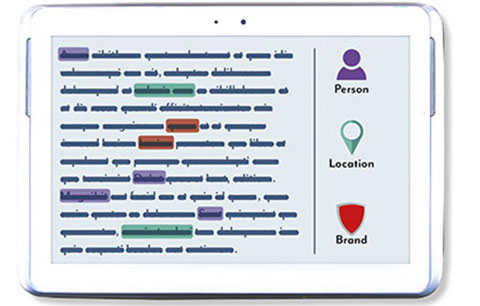
Named Entity Recognition (NER)
It consists of detecting single or multiple entities and associating them with pre-defined categories such as places, dates, people’s names, brands, percentages, monetary values, times or organizations.
NER helps identify the key elements in a text, so it is the base to sort unstructured data and detect important information such us categorizing tickets in customer support, gain insights from customer feedback, content recommendation, summaries, news, etc.
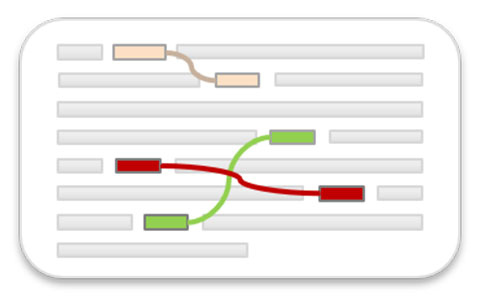
Semantic Annotation
This type of annotation includes from simple labeling of concepts and entities to relationships between words and phrases (dependency and coreference resolution), or dialogue annotation.
The annotations allow artificial intelligence algorithms perform tasks such as classifying, linking, inferencing, searching, or filtering.
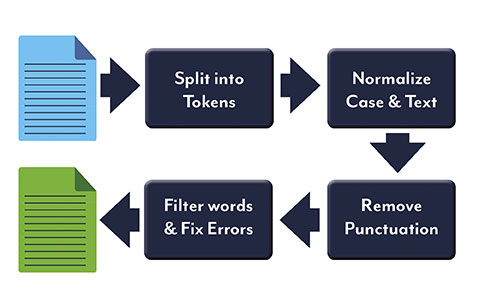
Text Cleansing
Raw text cannot be used directly to train machine learning or deep learning models. Instead, text must be cleaned. The process of cleansing depends on what the text is going to be used for.
It includes transforming the text into standard form (for example, yeeeah would be converted into its standard form yeah, numbers would be represented with words), and removing html/xml tags, characters which do not provide information, etc.
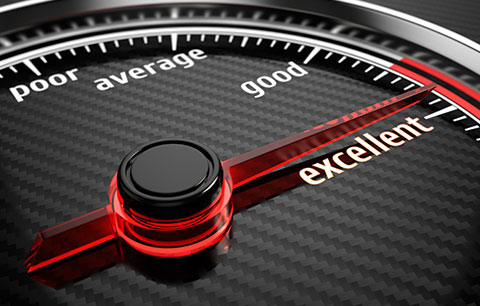
Text Validation
It consists of assessing the quality of a text dataset. The quality parameters to be measured depend on what the text dataset is going to be used for. For example, if the text is going to be used to train a sentiment analysis system, emoticons and punctuation symbols such as exclamation and question marks play an important role.
Text validation also includes assessing the quality of the labels and metadata associated to a dataset.
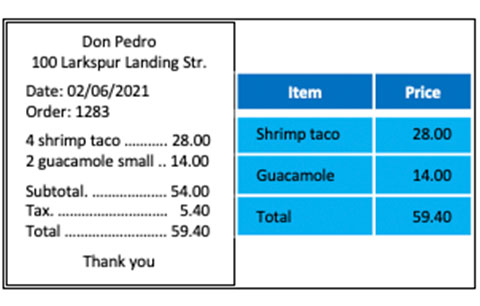
Receipt / Invoice Transcription
Training machine and deep learning systems able to interpret receipts, invoices, purchase orders, etc. requires the creation of datasets in which the different components of these documents are perfectly identified, marked and transcribed.
Sigma also provides this service for companies that want to externalize the generation of expense reports, automate the invoice processing, etc.