Facts in Data Annotation
YOUR AI IS ONLY AS GOOD AS ITS TRAINING DATA
High quality data is essential for developing unique and successful AI-based products and services.
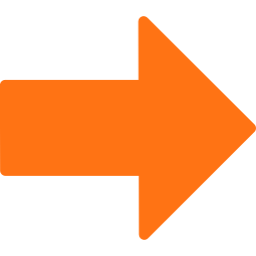
The production of high quality data at scale requires the best team, technology and tools. In particular:
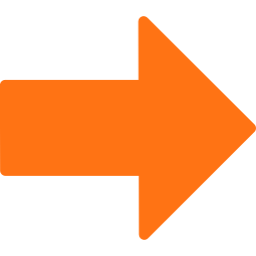
- Comprehensive QA methodology
- Work-flow oriented Intuitive tools
- ML-Assisted & Automation tools
- No crowd-sourcing
- Team created for the project
About 80% of the resources invested in the development of new AI-based solutions correspond to data annotation.
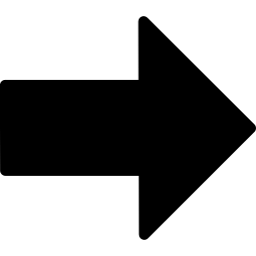
Annotation tools and procedures have to be designed to save time and resources:
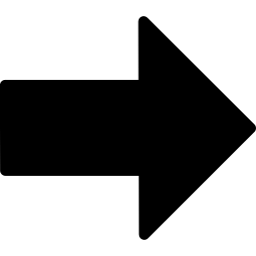
- Adapted User Interfaces
- Optimized work-flows, no latencies
- Active error minimization
- ML-Assisted tools
What is a comprehensive QA methodology?
The production of high-quality training data requires human intervention at some level. Therefore, when it comes to creating massive amounts of training data, achieving high quality is in the end, a human factor issue. It means that understanding what humans are good at and the sources of errors is crucial to designing the work procedures, the tools, and the QA methodologies.
Therefore, a comprehensive QA methodology is a QA methodology that is applied to the whole process: from the personnel’s selection and training to the quality metrics calculation. A comprehensive QA methodology includes preventive measures, ensuring the minimization of the number of errors, reactive measures to detect and correct mistakes once they have occurred, and metrics to obtain objective quality measures. It also includes specific QA tools that help optimize the QA resources and perform consistent QA assessments. A comprehensive approach to quality assessment reduces the time and resources needed to achieve the quality goals, and contributes significantly to the affordability of the data collection and annotation projects.
What is a work-flow oriented intuitive tool?
When users see an intuitive tool, they know exactly how to use it. They do not need to think of it, read manuals, experiment, or ask others. However, the fact that a tool is intuitive does not necessarily mean that it is useful, efficient or the best for a specific task.
Sigma created the concept of flow-oriented intuitive tool to refer to tools that, apart from being intuitive and user-friendly, have been optimized to perform a specific task (for example, audio transcription or image semantic annotation). This means that the interaction model of the user interface helps users to follow the optimal work-flow.
What are ML-Assisted & automation tools?
Sigma is using automation tools for pre- and post-processing to support human annotators. Technologies such as digital signal processing or machine learning can convert raw data into clean datasets ready to be annotated. It does not replace or reduce data labeling, but it improves the annotation throughput.
Similarly, machine learning assisted tools provide an order of magnitude improvement for many components of the annotation pipeline, including the annotation itself, to alleviate inefficiencies and free up human time. This equates to higher quality, higher throughput, and lower cost.
What is the difference between crowd-sourcing and creating the team for the project?
Sigma relies on highly trained human annotators instead of crowdsourcing the data annotation work. Crowdsourcing usually provides lower levels of quality, and it requires extensive quality assessment (QA) methods. Human annotators with the right profile and experience reduce the overall cost of the project and speed up the availability of annotated data because of the increased throughput and the time savings in QA.
Each data collection or annotation project has its specific characteristics, and therefore the selection of the right team is vital to guarantee an efficient achievement of the project goals. Sigma selects the team based on the skills and knowledge of its vetted candidates as well as on their previous experience in similar projects.
High quality human annotation is not only a matter of training and experience. It requires candidates with a very specific profile. For example, but not only, annotators who pay great attention to detail and are very patient in the annotation process. This is why Sigma does not crowdsource, but selects candidates with very particular profiles and skills, and trains them to produce consistent and high-quality annotations. Please, read our white paper on ML-assisted data annotation to learn more.
What is an adapted user interface?
Though most annotation user interfaces are similar, since each annotation project has its own characteristics, it is important to adapt the user interface to the annotation project. This is crucial in large annotation projects, where even small time savings can make a huge difference in terms of time and resources. Sigma always adapts the UI to the project at no cost for its clients.
An adapted UI is an UI that has been specifically designed to optimize, in a comprehensive way, the data annotation process in a project. The adapted user interface complies with a number of requirements such as:
- Work-flow oriented and intuitive: It has been designed to perform a specific task (for example, 3D bounding boxes, named entity recognition or audio transcription), so that the UI helps annotators follow the optimal sequence of actions in an intuitive and user-friendly way.
- Active error minimization: The UI includes automatic quality checks to prevent occasional and systematic annotation errors as well as to prevent annotators from accidentally skipping some annotation action.
How do optimized work-flows and no latencies help data annotation?
Annotation is the last step of a process that starts with raw data. How the raw data is received, stored and cleaned. How cleaned data is pre-processed and prepared to be assigned to the annotators. And, once the data is ready for annotation, how the data is assigned to each annotator, how the productivity is going to be monitored, when and how the data is reviewed to check quality, detect quality issues and design strategies to resolve them, etc. are some of the aspects that need to be designed and organized.
A proper organization of the work-flow, that uses the right human and technology resources in the right order and at right time, saves a significant amount of time and resources. Automation , when applicable, speeds up processes, reduces errors and cost, and helps human annotators focus attention where is most needed.
In addition to this, tools and processes must support the expected intense flow of data with no latencies, so there is no downtime and the annotated data throughput is the highest it can be.
How does active error minimization work?
The quality of the annotation depends on the type of preventive and reactive measures that are implemented, as well as on the metrics that are used to measure the quality level.
Preventive measures are the ones that prevent annotation errors from happening, such as selecting the right annotation team, providing annotators with the right training program, or keeping a continuous communication with the client to detect any issue that may arise quicky.
Reactive measures detect and fix errors and issues once they have occurred. There are three categories of errors and issues: occasional errors, systematic errors and interpretation issues, being the last two responsible for the lack of consistency of the annotated data.
Active error minimization is a preventive measure that includes a number of automatic quality checks which are implemented in the annotation user interface. They prevent occasional and systematic annotation errors from happening as well as prevent annotators from accidentally skipping some annotation action.